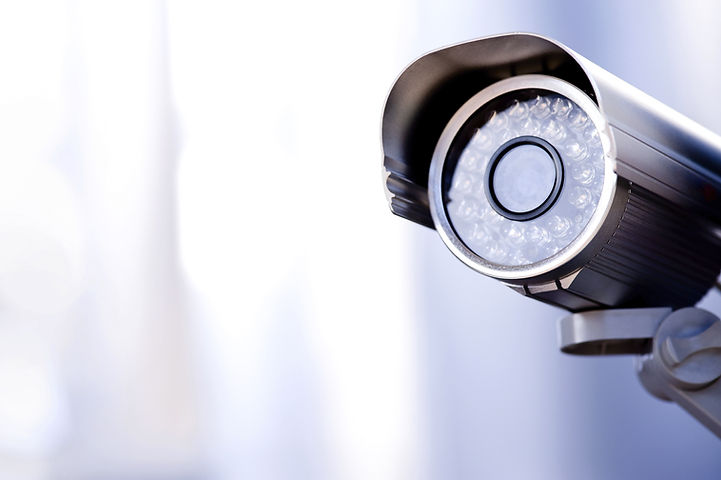
VISUAL UNDERTANDING
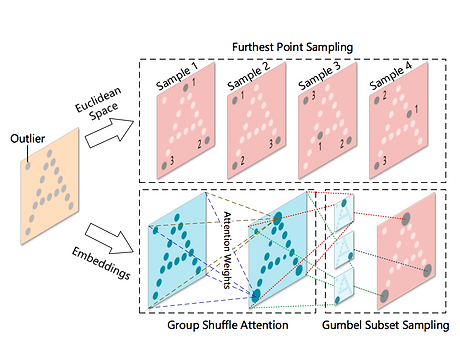
MODELING POINT CLOUDS WITH SELF-ATTENTION AND GUMBEL SUBSET SAMPLING
We demonstrate its ability to process size-varying inputs, and prove its permutation equivariance. Besides, prior work uses heuristics dependence on the input data (e.g., Furthest Point Sampling) to hierarchically select subsets of input points. Thereby, we for the first time propose an end-to-end learnable and task-agnostic sampling operation, named Gumbel Subset Sampling (GSS), to select a representative subset of input points.
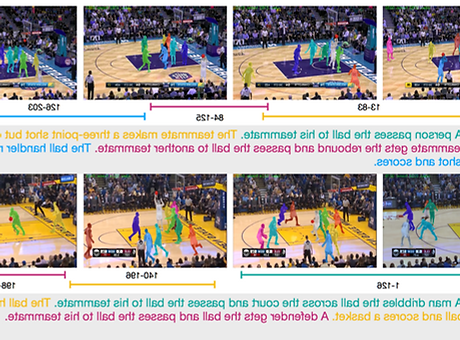
FINE-GRAINED VIDEO CAPTIONING FOR SPORTS NARRATIVE
This work makes the following contributions. First, to facilitate this novel research of fine-grained video caption, we collected a novel dataset called Fine-grained Sports Narrative dataset (FSN) that contains 2K sports videos with ground-truth narratives from YouTube.com. Second, we develop a novel performance evaluation metric named Fine-grained Captioning Evaluation (FCE) to cope with this novel task.
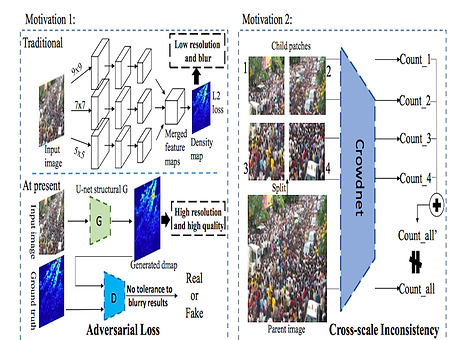
We propose a novel crowd counting (density estimation) framework called Adversarial Cross-Scale Consistency Pursuit (ACSCP). On one hand, a U-net structural network is designed to generate density map from input patch, and an adversarial loss is employed to shrink the solution onto a realistic subspace, thus attenuating the blurry effects of density map estimation.
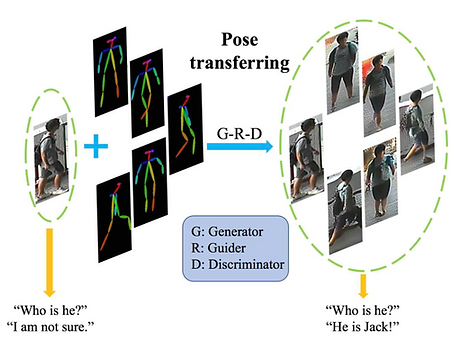
POSE TRANSFERRABLE PERSON RE-IDENTIFICATION
We propose a pose-transferrable person ReID framework which utilizes pose-transferred sample augmentations (i.e., with ID supervision) to enhance ReID model training. We also propose a novel guider sub-network which encourages the generated sample (i.e., with novel pose) towards better satisfying the ReID loss (i.e., cross-entropy ReID loss, triplet ReID loss).

We develop a novel Scale-Transferrable Detection Network (STDN) for detecting multi-scale objects in images. The proposed network is equipped with embedded super-resolution layers to explicitly explore the interscale consistency nature across multiple detection scales.

Despite recent emergence of adversarial based methods for video prediction, existing algorithms often produce unsatisfied results in image regions with rich structural information (i.e., object boundary) and detailed motion (i.e., articulated body movement). To this end, we present a structure preserving video prediction framework to explicitly address above issues and enhance video prediction quality
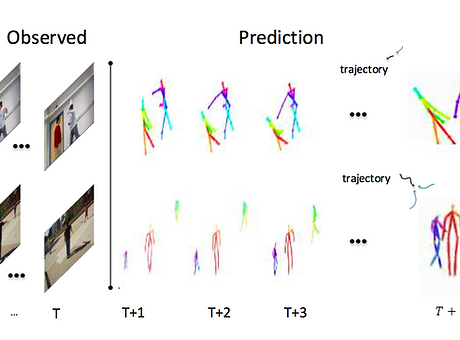
CVPR'2018
We propose a multi- granularity interaction prediction network which integrates both global motion and detailed local action. Built on a bi- directional LSTM network, the proposed method possesses between granularities links.
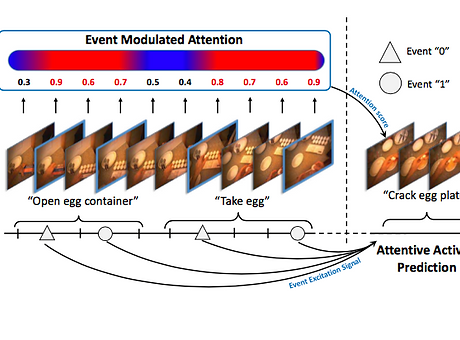
EGOCENTRIC ACTIVITY PREDICTION VIA EVENT MODULATED ATTENTION
ECCV'2018
We develop Point Attention Transformers (PATs), using a parameter-efficient Group Shuffle Attention (GSA) to replace the costly Multi-Head Attention. We demonstrate its ability to process size-varying inputs, and prove its permutation equivariance.