
AI300
SJTU Vision and Learning Lab
Exploiting the Essence of Learning and Vision
Department of Electronic Engineering, Shanghai Jiao Tong University, Shanghai, China 200240
LEARNING CONTEXT GRAPH FOR PERSON SEARCH
CVPR'2019 Oral
Person re-identification has achieved great progress with deep convolutional neural networks. However, most previous methods focus on learning individual appearance feature embedding, and it is hard for the models to handle difficult situations with different illumination, large pose variance and occlusion. In this work, we take a step further and consider employing context information for person search. For a probe-gallery pair, we first propose a contextual instance expansion module, which employs a relative attention module to search and filter useful context information in the scene. We also build a graph learning framework to effectively employ context pairs to update target similarity. These two modules are built on top of a joint detection and instance feature learning framework, which improves the discriminativeness of the learned features. The proposed framework achieves state-of-the-art performance on two widely used person search datasets.
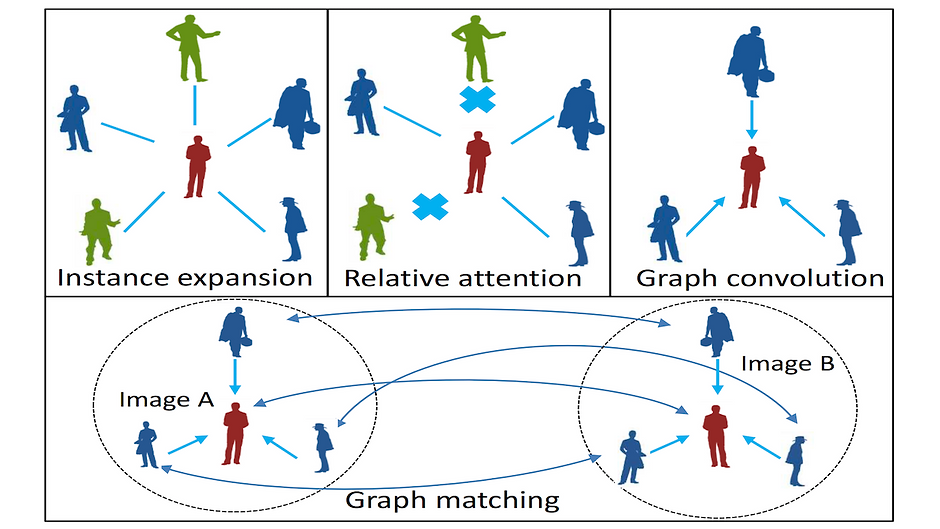
3D DEEP LEARNING FROM CT SCANS PREDICTS TUMOR INVASIVENESS OF SUBCENTIMETER PULMONARY ADENOCARCINOMAS
Cancer Reseach
Identification of early-stage pulmonary adenocarcinomas before surgery, especially in cases of subcentimeter cancers, would be clinically important and could provide guidance to clinical decision making. In this study, we developed a deep learning system based on 3D convolutional neural networks and multitask learning, which automatically predicts tumor invasiveness, together with 3D nodule segmentation masks. The system processes a 3D nodule-centered patch of preprocessed CT and learns a deep representation of a given nodule without the need for any additional information. A dataset of 651 nodules with manually segmented voxel-wise masks and pathological labels of atypical adenomatous hyperplasia (AAH), adenocarcinomas in situ (AIS), minimally invasive adenocarcinoma (MIA), and invasive pulmonary adenocarcinoma (IA) was used in this study. We trained and validated our deep learning system on 523 nodules and tested its performance on 128 nodules. An observer study with 2 groups of radiologists, 2 senior and 2 junior, was also investigated. We merged AAH and AIS into one single category AAH-AIS, comprising a 3-category classification in our study. The proposed deep learning system achieved better classification performance than the radiologists; in terms of 3-class weighted average F1 score, the model achieved 63.3% while the radiologists achieved 55.6%, 56.6%, 54.3%, and 51.0%, respectively. These results suggest that deep learning methods improve the yield of discriminative results and hold promise in the CADx application domain, which could help doctors work efficiently and facilitate the application of precision medicine.
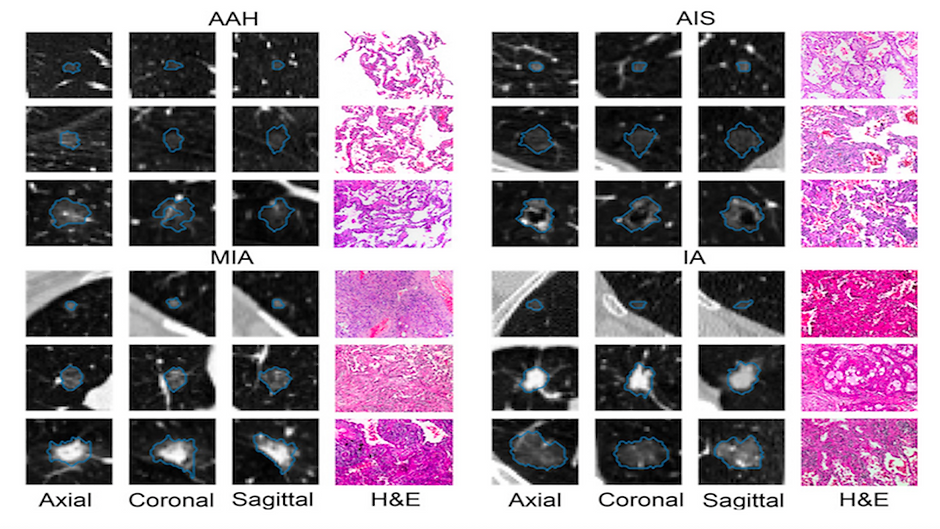
ARTISTNET
We propose a neural painter framework based on multi-agent reinforcement learning, to generate stroke-based style-transferred oil paintings. The proposed framework includes two agents, a reconstruction agent deciding reconstructing parameters of strokes and a style agent choosing the style parameters. Given content images, our framework creates stroke-based oil paintings in a certain artist’s style, which can be further rendered by real robots.
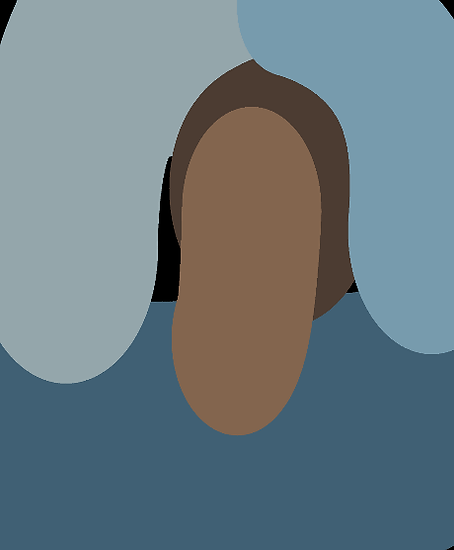
RECURRENT MODELING OF INTERACTION CONTEXT FOR COLLECTIVE ACTIVITY RECOGNITION
CVPR'2017
Modeling of high order interactional context, e.g., group interaction, lies in the central of collective/group activity recognition. However, most of the previous activity recognition methods do not offer a flexible and scalable scheme to handle the high order context modeling problem. To explicitly address this fundamental bottleneck, we propose a recurrent interactional context modeling scheme based on LSTM network. By utilizing the information propagation/aggregation capability of LSTM, the proposed scheme unifies the interactional feature modeling process for single person dynamics, intra-group (e.g., persons within a group) and inter-group(e.g., group to group)interactions. The proposed high order context modeling scheme produces more discriminative/descriptive interactional features. It is very flexible to handle a varying number of input instances (e.g., different number of persons in a group or different number of groups) and linearly scalable to high order context modeling problem. Extensive experiments on two benchmark collective/group activity datasets demonstrate the effectiveness of the proposed method.
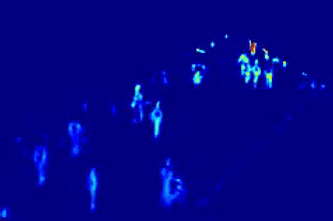
VIDEO PREDICTION VIA SELECTIVE SAMPLING
NerIPS'2018
Most adversarial learning based video prediction methods suffer from image blur, since the commonly used adversarial and regression loss pair work rather in a competitive way than collaboration, yielding compromised blur effect. In the meantime, as often relying on a single-pass architecture, the predictor is inadequate to explicitly capture the forthcoming uncertainty. Our work involves two key insights: (1) Video prediction can be approached as a stochastic process: we sample a collection of proposals conforming to possible frame distribution at following time stamp, and one can select the final prediction from it. (2) De-coupling combined loss functions into dedicatedly designed sub-networks encourages them to work in a collaborative way. Combining above two insights we propose a two-stage framework called VPSS (Video Prediction via Selective Sampling). Specifically aSampling module produces a collection of high quality proposals, facilitated by a multiple choice adversarial learning scheme, yielding diverse frame proposal set. Subsequently a Selection module selects high possibility candidates from proposals and combines them to produce final prediction. Extensive experiments on diverse challenging datasets demonstrate the effectiveness of proposed video prediction approach, i.e., yielding more diverse proposals and accurate prediction results.
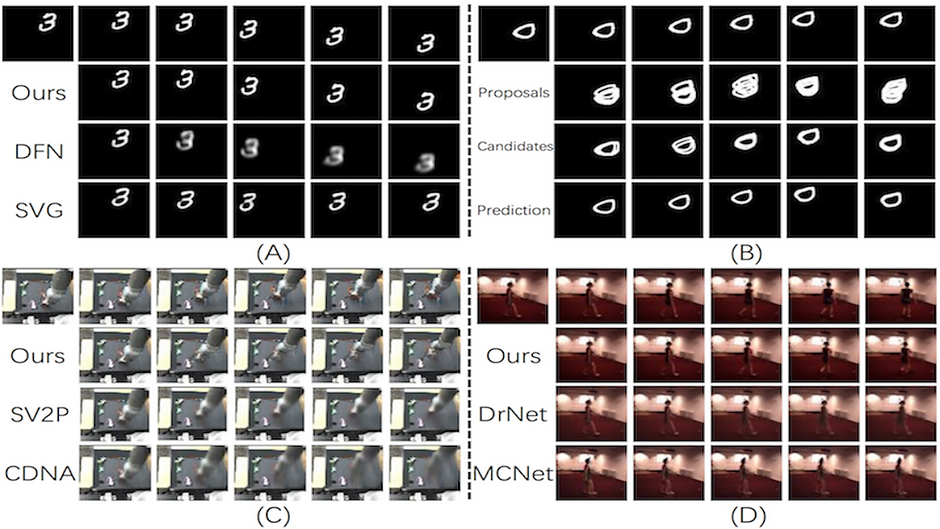
CONTACT
No. 300, Building 5, School of Electronic Information and Electrical Engineering, Shanghai Jiao Tong University
021-34204522